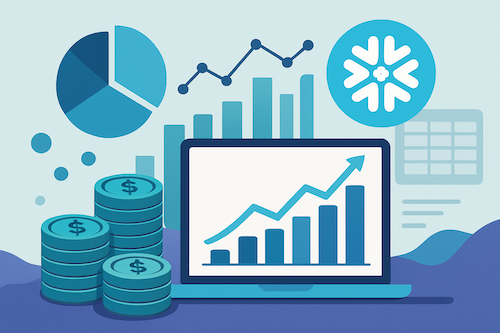
Table of Contents
The Evolving Data Landscape in Finance
Finance departments today often find themselves wrestling with data. Traditional systems frequently lead to frustrating data silos, scalability headaches, and reporting processes that move at a glacial pace. It’s a common pain point: critical financial insights buried under layers of inefficiency. The modern enterprise, however, demands agility. This pressing need has paved the way for the modern data stack, emphasizing platforms that are not only scalable and governed but also remarkably flexible. Observations from numerous system assessments suggest that Snowflake is rapidly emerging as a pivotal enabler in this financial transformation. This piece delves into Snowflake’s architecture, its distinct advantages for financial analytics, robust integration strategies, key use cases, and important implementation considerations.
Understanding Snowflake’s Architecture: Why It Matters for Finance
So, what makes Snowflake tick, and why is its architecture particularly beneficial for the financial domain? A perspective forged through years of navigating real-world enterprise integrations highlights several core strengths. Its decoupled storage and compute design is fundamental. This allows finance teams to scale resources independently – ramping up for peak periods like month-end close and scaling down to manage costs effectively, all while ensuring performance consistency for varied concurrent workloads.
Then there are multi-cluster virtual warehouses. Imagine distinct operational lanes for different finance functions – FP&A, regulatory reporting, risk management – each with dedicated resources, thereby eliminating contention and helping to secure SLAs for vital financial processes. This architectural choice directly addresses the historical bottlenecks many finance departments have faced. Furthermore, Snowflake’s centralized storage layer champions a single source of truth, adeptly handling both structured (think ERP data) and semi-structured data (like JSON feeds or CSVs). This capability is crucial for breaking down silos and simplifies access to diverse financial datasets, including market or alternative data often found in the Snowflake Marketplace. This can foster secure, governed data sharing without the typical friction of data movement. Finally, its cloud services layer takes on the heavy lifting of transaction management, security, metadata, and query optimization, significantly reducing administrative burdens for finance IT.
Key Snowflake Capabilities Powering Financial Analytics & Operations
Beyond its architecture, specific Snowflake features are significantly boosting financial analytics. The platform’s scalability and performance are paramount, especially when dealing with complex financial reports and extensive historical data for trend analysis. Many organizations report a marked acceleration in their reporting cycles.
A critical area where Snowflake shines is in data integration and ERP data consolidation. Insights distilled from numerous complex system deployments indicate that a unified financial view is a common strategic goal. Snowflake facilitates this by efficiently ingesting data from a multitude of sources – SAP, NetSuite, Oracle, various CRM and HR systems, and even legacy databases – using tools like Snowpipe for continuous loading or various ELT solutions for batch processing. The ability to consolidate data from disparate ERP instances into a single, coherent view is a game-changer for many.
Naturally, for finance, enhanced security and governance are non-negotiable. Snowflake addresses this with robust features like Role-Based Access Control (RBAC), column-level security, row-level access policies, and dynamic data masking for sensitive information. These tools are instrumental in meeting stringent regulatory demands (think SOX, GDPR, CCPA) and providing clear audit trails. Moreover, its support for advanced analytics and AI/ML, through capabilities like Snowpark (allowing Python, Java, or Scala code to run directly within Snowflake), opens doors for sophisticated use cases such as fraud detection, credit risk scoring, and AI-powered forecasting. The platform’s Time Travel and Zero-Copy Cloning features also offer practical benefits like point-in-time reporting and cost-effective development environments without impacting production.
Real-World Financial Use Cases with Snowflake
The practical applications of Snowflake in finance are diverse and impactful. We’ve seen how it significantly accelerates financial and enterprise reporting, enabling interactive dashboards for KPIs, variance analysis, and profitability deep dives. For Financial Planning & Analysis (FP&A) teams, it means better integration of actuals with budgets and forecasts, often augmented by AI/ML for more accurate predictive insights.
In risk management and regulatory compliance, Snowflake’s capacity to analyze vast datasets aids in critical functions like fraud detection and anti-money laundering (AML) activities, while also streamlining regulatory reporting. Another common pattern is ERP data consolidation and modernization, where data from aging ERP systems is moved to Snowflake to create that much-needed unified view, simplifying audits and unlocking advanced analytics previously out of reach. Some organizations even leverage it for cloud spend management and FinOps, analyzing cloud costs (including Snowflake’s own) for optimization.
Integrating Snowflake with the Enterprise Financial Ecosystem
Snowflake doesn’t exist in a vacuum; its power is amplified by how it connects with the broader enterprise financial ecosystem. When connecting to core financial systems (ERPs, CRMs), organizations typically employ ELT/ETL tools like Fivetran, Matillion, or dbt, or develop custom data pipelines, often involving API integration strategies.
For BI and visualization, native connectors to tools such as Tableau, Power BI, and Looker are crucial for translating raw data into actionable financial dashboards. Similarly, integration with data science and ML platforms like Dataiku or H2O.ai, or cloud-native ML services, allows finance teams to build and deploy sophisticated models. Throughout this integration process, a consistent focus on data consistency and quality is, of course, paramount.
Strategic Implementation Considerations for Finance
Successfully adopting Snowflake in a financial context requires careful planning. A well-thought-out data migration strategy is key when moving from legacy systems. From the outset, establishing a robust data governance framework – defining roles, policies, and processes specifically for financial data within Snowflake – is essential. This isn’t just a technical task; it’s a foundational business requirement.
Cost optimization is another critical area. This involves right-sizing virtual warehouses, fine-tuning queries, and managing storage efficiently by understanding Snowflake’s pricing model and using its resource monitors. What about the team? Developing or acquiring skills in data engineering, analytics, and governance, either within the finance team or through close collaboration with IT, is often necessary. Don’t underestimate change management; ensuring finance users can, and do, leverage the new capabilities is vital for realizing the platform’s full potential.
The Future: Snowflake and the Next Generation of Financial Analytics
Looking ahead, Snowflake continues to evolve, with emerging features poised to further impact financial analytics. Think of capabilities like Hybrid Tables for transactional workloads, Streamlit integration for building financial applications directly within Snowflake, Snowpark Container Services, and Snowflake Cortex for accessible AI. The trend is clearly towards real-time financial insights and a more deeply data-driven finance function. Snowflake appears well-positioned to play a significant role in enabling what many are calling the “AI Data Cloud” for finance departments, transforming how financial data is managed and leveraged.
Charting Your Course
The journey to modernize financial data infrastructure is unique for every enterprise. Yet, the consistent themes of needing scalability, robust performance, stringent governance, and advanced analytical capabilities resonate across the board. Platforms like Snowflake are offering compelling answers to these long-standing challenges, acting as a strategic asset rather than just a data repository. How might such a platform reshape your financial data strategy? It’s certainly a question worth exploring.
For further discussion on leveraging technology for financial transformation, feel free to connect with me on LinkedIn.