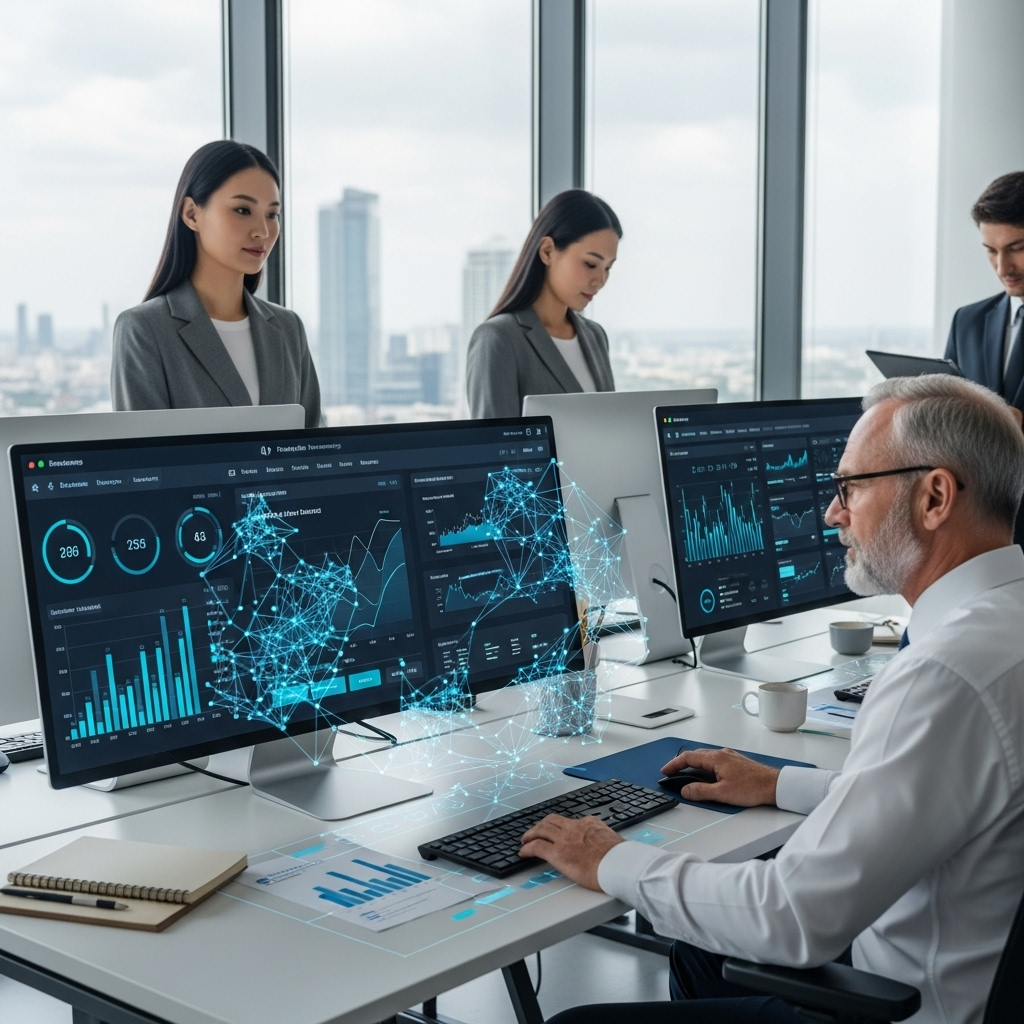
Table of Contents
Note: This article focuses specifically on AI applications within Financial Planning and Analysis (FP&A) functions. For a broader examination of AI in financial analysis, see our related article on foundational concepts.
Financial Planning and Analysis (FP&A) functions face mounting pressure to deliver more accurate forecasts, deeper insights, and faster analysis. Artificial intelligence applications offer transformative capabilities that address these challenges, moving beyond simple automation to deliver genuine analytical enhancements. What specific AI applications are creating the most impact in financial planning? It’s a rapidly evolving field.
Predictive Forecasting
Predictive forecasting represents one of AI’s most immediately valuable applications. Traditional forecasting methods, including time series analysis, driver-based models, or analogous estimations, all suffer from limited pattern recognition capabilities and human biases. Machine learning models trained on historical financial data can identify complex relationships between operational metrics and financial outcomes that traditional approaches might miss. The most effective implementations combine multiple prediction models, each specialized for different aspects of the forecast (revenue streams, expense categories, etc.), which are then aggregated into comprehensive projections. This ensemble approach typically delivers a 15-30% improvement in forecast accuracy compared to traditional methods. That’s a significant uplift.
Anomaly Detection
Anomaly detection capabilities enhance financial control environments. AI systems continuously monitor transaction patterns, flagging unusual activities that might indicate errors, fraud, or emerging business issues. Unlike rule-based detection systems, machine learning approaches adapt to evolving business patterns without constant reconfiguration. Organizations implementing these systems report both efficiency benefits (reduced manual review requirements) and effectiveness improvements (earlier detection of significant variances). The most sophisticated implementations incorporate feedback loops where analyst determinations about flagged items continuously improve the detection algorithm’s accuracy. It’s like having an ever-vigilant digital auditor.
Scenario Modeling
Scenario modeling receives significant enhancement through AI applications. Traditional scenario planning typically examines a limited number of alternative futures based on manual parameter adjustments. Modern AI approaches enable Monte Carlo simulations that generate thousands of potential scenarios by simultaneously varying multiple business drivers. These comprehensive simulations provide more robust risk assessments and identify non-obvious interactions between different business factors. Finance teams leverage these capabilities to develop more resilient strategies that perform adequately across a wider range of potential futures rather than optimizing for a single expected scenario. Don’t you think this provides better preparedness?
Natural Language Processing (NLP)
Natural language processing (NLP) transforms both inputs to and outputs from financial planning processes. On the input side, NLP systems extract relevant financial implications from unstructured data sources like earnings calls, industry reports, supplier communications, and social media. These insights supplement traditional structured data, providing earlier indicators of market shifts. On the output side, NLP generates narrative explanations of financial results and variances, translating complex analytical findings into clear business language. These capabilities significantly enhance the accessibility of financial insights beyond specialized finance teams.
Cash Flow Optimization
Cash flow optimization models employ reinforcement learning techniques to improve working capital management. These systems analyze historical payment patterns, seasonal fluctuations, and vendor/customer behaviors to recommend optimal timing for payables, collections efforts, and short-term investment decisions. Organizations implementing these capabilities report both efficiency benefits from automated analysis and effectiveness improvements through more precise cash positioning. The most sophisticated applications incorporate real-time data streams to continuously update recommendations as conditions change. It’s about making your cash work smarter, not just harder.
Intelligent Process Automation
Intelligent process automation (IPA) extends beyond basic RPA to incorporate cognitive capabilities that handle exceptions and unstructured inputs. In financial planning contexts, these systems automate data collection, reconciliation, and adjustment processes with minimal human intervention. Unlike traditional automation that requires strictly defined processes, AI-enhanced automation adapts to variations in input formats, identifies and resolves data inconsistencies, and learns from human handling of exceptions. This adaptability proves particularly valuable for financial planning processes that incorporate data from multiple systems with evolving formats.
Driver Identification Algorithms
Driver identification algorithms uncover non-obvious relationships between operational metrics and financial outcomes. Rather than relying on finance teams to hypothesize potential drivers, these systems analyze hundreds of operational variables to identify those with the strongest predictive relationship to financial results. This data-driven approach often uncovers unexpected connections, such as specific customer service metrics that strongly predict retention rates or supply chain measurements that lead revenue growth by several quarters. These insights enable more responsive planning models that incorporate leading indicators rather than relying solely on trailing financial metrics.
Recommendation Engines
Recommendation engines suggest specific actions to improve financial performance based on pattern recognition across large datasets. These systems identify successful tactics from historical data or peer comparisons, then recommend similar approaches when analogous conditions appear. For example, recognizing that specific pricing strategies succeeded in similar market conditions, or that particular cost control measures effectively addressed similar margin pressures in the past. These recommendations transform planning from a reporting exercise into an action-oriented process directly supporting decision-making.
Adoption Approaches
Adoption approaches vary significantly among organizations. Those achieving the greatest success typically start with focused applications addressing specific high-value planning challenges rather than comprehensive AI transformations. These targeted implementations build both technical capabilities and organizational trust before expanding to more complex applications. Successful programs also emphasize partnership between finance teams and data scientists, ensuring that AI models incorporate appropriate financial domain knowledge while leveraging advanced analytical techniques. It’s a journey, not a destination.
For professional connections and further discussion, find me on LinkedIn.