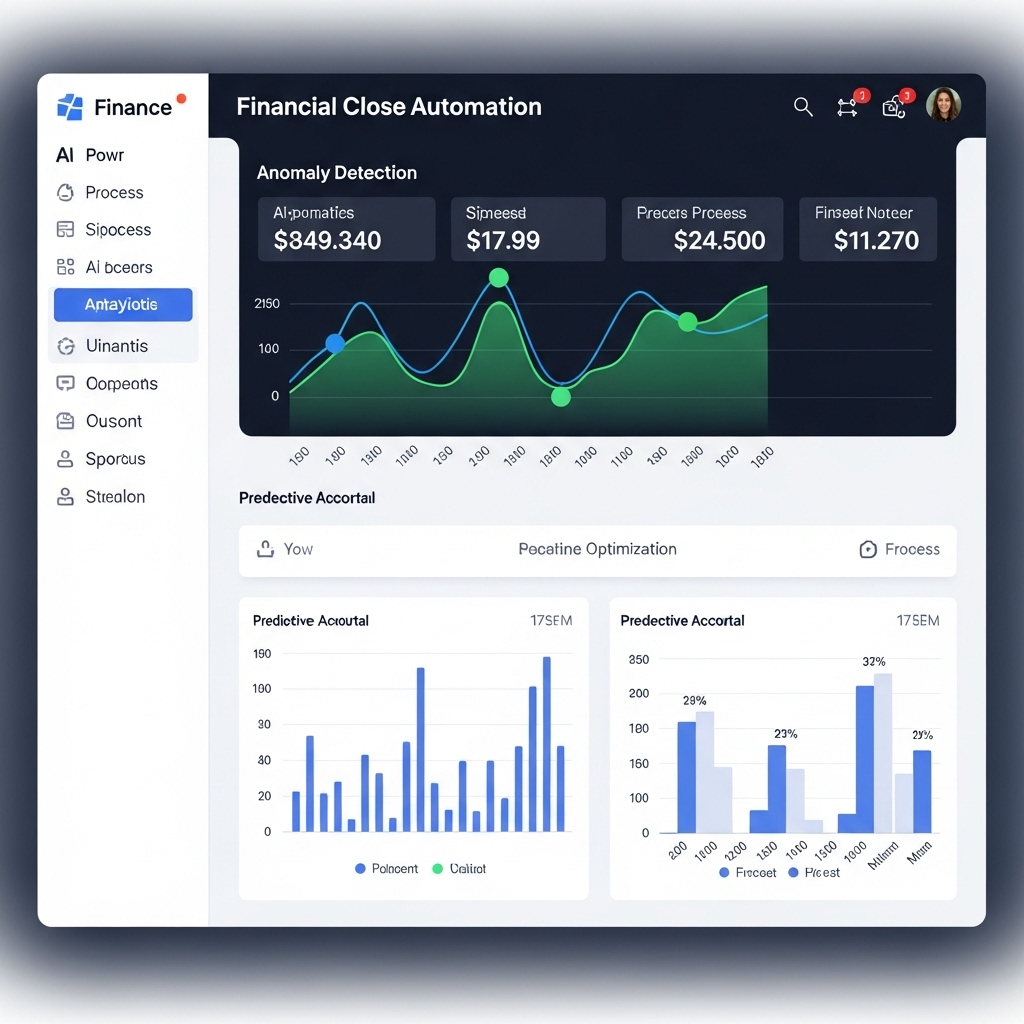
Table of Contents
Financial Close Automation: Beyond the First Wave
The financial close process has undergone significant transformation in recent years. Most mid-to-large organizations have implemented some form of close automation, typically focused on workflow management, task scheduling, and basic reconciliation matching. This first wave delivered meaningful efficiency gains but still relies heavily on predefined rules and human judgment for exceptions.
A second transformation wave now emerges through AI augmentation of the close process. This represents a fundamental shift from rule-based automation to predictive, adaptive systems that continuously improve through operational feedback loops.
The Intelligent Close Ecosystem
AI capabilities enhance multiple dimensions of the close process simultaneously. Analysis of recent implementations reveals four primary application categories:
Anomaly Detection and Investigation The most mature AI use case applies machine learning to identify unusual transactions requiring investigation. Unlike traditional rule-based exception flagging, these systems detect subtle pattern deviations that might indicate errors or opportunities for process improvement.
Reconciliation Intelligence Advanced machine learning models now match transactions with success rates exceeding 95% even with imperfect data. These systems process unstructured information from multiple sources, extract relevant data points, and propose matches based on probabilistic reasoning rather than rigid rules.
Forecasting and Accrual Optimization Predictive analytics models increasingly support the accrual process. By analyzing historical patterns and current activity indicators, these systems generate highly accurate accrual recommendations, reducing both estimation effort and subsequent true-up adjustments.
Close Process Optimization Perhaps most intriguing, process mining and machine learning combine to analyze the close workflow itself. These tools identify bottlenecks, predict potential delays, and recommend process adjustments to optimize the close timeline.
Implementation Architecture Considerations
Organizations implementing AI-augmented close capabilities face critical architectural decisions. Market analysis points to three primary models. First, leveraging native AI capabilities within financial systems, where major ERP and financial management platforms embed AI functions directly. This offers straightforward integration but might lack the advanced features of specialized providers. Second, using dedicated close management platforms with embedded AI; these deliver purpose-built functionality but can pose integration challenges. Third, creating coordinated ecosystems with specialized AI components, allowing for best-of-breed tools but increasing architectural complexity. Each model has its merits, though my observations suggest complex, multi-system environments often benefit most from the ecosystem approach, despite integration hurdles. What’s particularly noteworthy here is how process mining and machine learning combine to analyze the close workflow itself. These tools identify bottlenecks, predict potential delays, and recommend process adjustments to optimize the close timeline.
Data Foundations for Intelligent Close Processes
The effectiveness of AI in the close process hinges on data quality and accessibility. My analysis shows that organizations reaping the greatest benefits consistently possess key data capabilities: a unified financial data repository for consistent, high-quality information; robust operational data integration linking financial transactions to business events; and diligent historical pattern preservation for longitudinal data learning. It’s a foundational truth, really: without these, AI initiatives often fall short, regardless of technological sophistication.
Human-AI Collaboration Models
Successful implementations establish thoughtful collaboration between finance professionals and AI. This involves transparent recommendation frameworks that clarify AI confidence levels and rationale, alongside feedback mechanisms enabling human decisions to refine future AI outputs. Furthermore, exception-based workflows should direct human expertise to areas requiring judgment, all supported by continuous improvement processes that analyze both AI and human performance. Such a collaborative model addresses adoption challenges and ensures appropriate governance.
Measuring Success Beyond Efficiency
While efficiency is key, evaluating AI-augmented close processes requires a broader view. A comprehensive framework also assesses risk reduction from improved anomaly detection, insight generation that uncovers process improvements, close quality as measured by post-close adjustment frequency, and resource alignment that shifts teams from mechanical tasks to analytical work. This balanced assessment ensures AI investments achieve their full potential.
The financial close process stands at an inflection point. Organizations thoughtfully implementing AI augmentation now will likely establish sustainable competitive advantages through superior financial intelligence. Those deferring these capabilities risk increasing competitive disadvantage as the technology adoption curve accelerates.