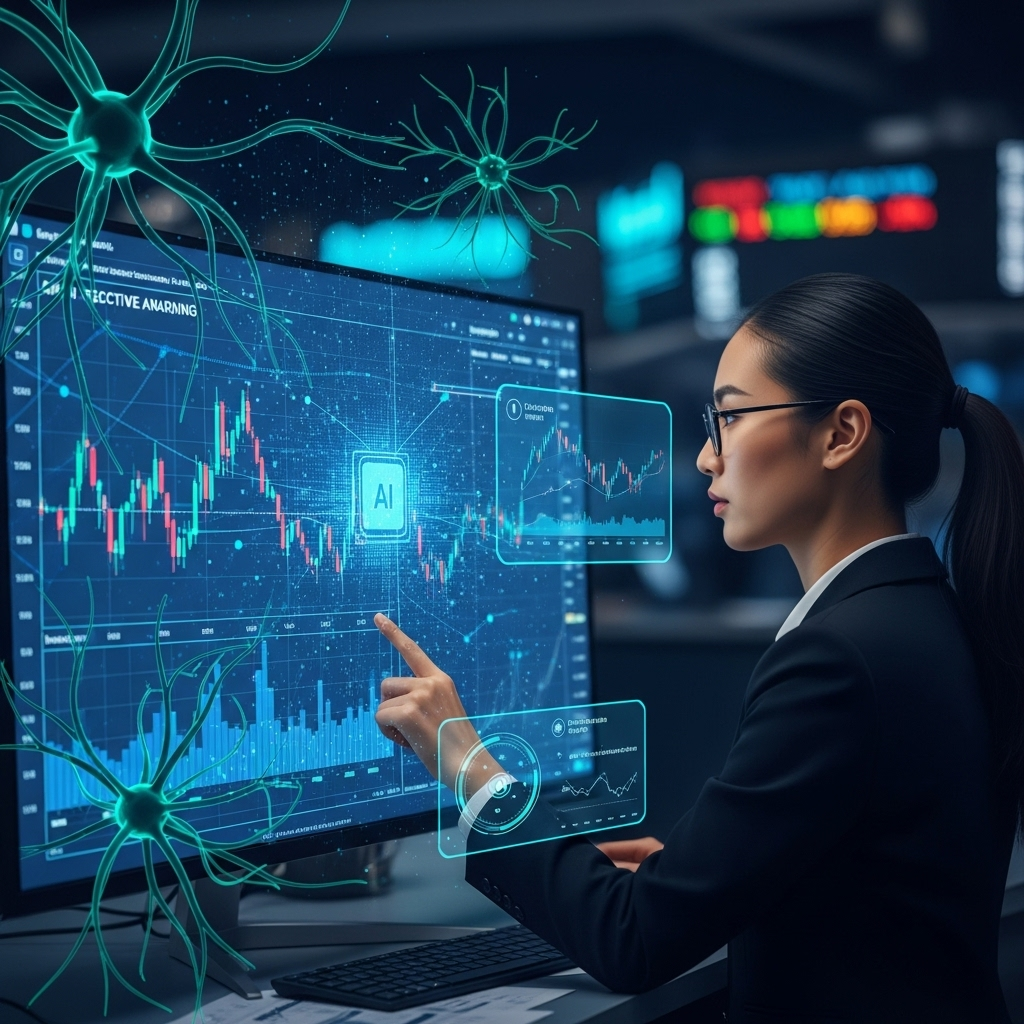
Table of Contents
The evolution of artificial intelligence in financial analysis now stretches far beyond merely automating repetitive tasks. It’s increasingly about how AI empowers and augments human financial analysts. This strategic shift involves leveraging advanced pattern recognition, bolstering predictive capabilities, and refining decision support mechanisms. We’re seeing practical implementation approaches emerge across the industry, showcasing AI’s tangible impact on the quality and depth of financial insight.
AI’s Augmentative Power Beyond Simple Tasks
AI’s true augmentative power shines when it moves past simple task execution. Consider its pattern recognition prowess; AI can unearth complex relationships and subtle correlations within vast datasets that traditional rule-based systems or even human analysts might easily miss. It identifies novel correlations and emerging anomalies, adapting dynamically as financial landscapes shift. This isn’t just about speed; it’s about achieving a new depth of understanding.
Predictive modeling also gets a significant boost. Instead of relying on static forecasts, AI models can continuously refine themselves with real-time data feeds, enhancing accuracy without requiring constant manual oversight. Furthermore, AI-driven scenario simulations can present analysts with a much wider array of computed alternatives and potential outcomes, pushing beyond the typical boundaries of human analysis. The integration of multimodal data (blending structured numerical information with unstructured text from news articles, regulatory filings, or earnings call transcripts) provides a richer, more contextualized analytical tapestry.
Highlighted Applications of Advanced AI Capabilities
Several applications highlight these advanced capabilities. Anomaly detection, for instance, now often comes with explainable results, a far cry from older systems that were frequently plagued by a high volume of false positives. Modern AI not only pinpoints subtle deviations from expected patterns but can also clarify its reasoning, allowing analysts to prioritize investigations much more efficiently.
Earnings quality assessment also benefits significantly, as AI systems scrutinize financial statements for nuanced indicators of potential manipulation or aggressive accounting practices, offering insights that once demanded deep forensic expertise. In credit evaluation, AI algorithms can incorporate a diverse range of alternative data sources, like utility payments or rental history, to identify creditworthy applicants who might be overlooked by traditional scoring models. Investment research, too, is transformed, with AI processing vast quantities of text to extract market sentiment, identify emerging themes, and provide a contextualized view that complements quantitative financial models. This allows for a more holistic approach to financial analysis, especially when dealing with complex global entities.
Keys to Successful Implementation
For successful implementation, a hybrid intelligence system, where human judgment strategically steers AI’s analytical power, often yields the best results. Analysts might define initial hypotheses or critical questions, with AI then exploring the data landscape and presenting findings for human synthesis and interpretation. Explainable AI (XAI) is also crucial, particularly in finance; providing transparency into model outputs and decision-making processes is a vital aspect when those decisions require justification or face regulatory review.
Continuous learning pipelines allow AI models to improve their performance progressively over time while maintaining stability and avoiding model drift. Federated learning is an emerging approach that addresses data privacy concerns by enabling models to be trained across distributed data sources without centralizing sensitive information, a key consideration in the financial sector.
Starting the Journey: Data Preparation
The journey to AI augmentation in finance often begins with tackling the perennial challenge of data preparation. It’s not uncommon for analysts to spend the bulk of their time (sometimes up to 80%) simply gathering, cleaning, and structuring data. Machine learning can accelerate this considerably. Automated anomaly detection during data ingestion, intelligent data matching algorithms, and adaptive classification models can significantly cut down this preparation time, often by 40-50% in observed cases. This, crucially, frees up analysts for higher-value work like interpretation and strategic thinking.
Excelling with Multi-Dimensional Pattern Recognition
AI also excels in multi-dimensional pattern recognition, moving far beyond simple variance checks against a budget. It can identify intricately correlated expense categories across different departments, subtle related seasonal revenue fluctuations influenced by external factors, or distinct customer behavior clusters that reveal underlying business dynamics. One manufacturing company, by way of example, leveraged this capability to find subtle but significant links between their equipment maintenance schedules, production quality metrics, and subsequent warranty costs. This insight led to optimized maintenance routines and a reported 14% reduction in those warranty-related costs.
Enhanced forecasting is another key area where AI delivers. By integrating diverse data inputs (such as macroeconomic indicators, specific industry trends, weather patterns for certain sectors, or even social media sentiment) AI models can achieve greater accuracy than traditional time-series extrapolations alone. A retail organization, for instance, was able to cut its forecast error by 28% through such an augmented approach, leading to demonstrably improved inventory management and reduced holding costs.
Unlocking Insights with Natural Language Processing (NLP)
Natural Language Processing (NLP) unlocks invaluable insights from the vast sea of unstructured text data. Imagine AI systems analyzing earnings call transcripts in near real-time for shifts in management tone or specific forward-looking statements. Or picture AI scanning thousands of pages of regulatory filings to identify evolving risk disclosures or new compliance obligations. This qualitative insight, previously very difficult to systematically incorporate and analyze at scale, enriches financial analysis profoundly.
The control environment within an organization also strengthens with AI. Advanced anomaly detection models can learn complex, multi-dimensional patterns of normal behavior, understand the context of transactions, and adapt continuously to changing business processes. This significantly improves fraud detection capabilities and can reduce the burden of false positives that often plague simpler rules-based systems. A global manufacturer, for instance, reported cutting its internal investigation workload by 62% while simultaneously boosting the identification rate of actual issues by 34% after implementing such an AI-driven system.
Enhancing Investment Analysis and Process Automation
For investment analysis, machine learning enhances scenario modeling by identifying non-linear relationships between variables and allowing for more sophisticated stress testing across multiple correlated factors. This leads to more robust and nuanced investment cases. Even traditional process automation gets smarter with AI; natural language generation (NLG) can now produce initial drafts of financial analysis reports, highlighting key variances, trends, and suggesting areas that warrant deeper human review. This shifts the analyst’s role from primarily report generation to strategic interpretation and advisory.
Best Practices for Technology Implementation
When implementing these advanced technologies, organizations often find the most success by starting with processes known for high friction or significant manual effort. Prioritizing augmentation of human analysts over outright replacement typically fosters better adoption and more effective outcomes. Establishing rapid feedback loops between the finance professionals and the data science teams is also critical. It’s about creating hybrid teams that blend deep financial expertise with data science acumen. Successful integration means embedding AI capabilities directly within existing finance functions and workflows, not isolating them in a separate R&D silo. This ensures that AI applications remain tethered to real business needs and deliver measurable value.
Future Capabilities and Emerging Trends
Looking ahead, the field is rapidly evolving. Capabilities like causal inference (which aims to distinguish true cause-and-effect relationships from mere correlations) and more advanced natural language generation for creating sophisticated financial narratives promise even deeper insights. Temporal graph analytics, which examines how financial relationships and networks (e.g., between entities, transactions, and individuals) evolve over time, will also offer powerful new perspectives for risk management and opportunity identification.
Paramount Considerations for AI in Finance
However, several considerations remain paramount as AI becomes more ingrained in finance. Data quality governance becomes even more critical; sophisticated AI algorithms can unfortunately produce plausible but dangerously wrong insights if fed flawed or biased data. Developing human capability (training analysts to collaborate effectively with AI tools and interpret their outputs critically) is essential. Furthermore, implementing robust ethical frameworks to address potential biases in data and models, and to ensure fairness and transparency, is not just a good idea, it’s a necessity. Regulatory compliance strategies must also adapt to this evolving technological landscape, ensuring that AI usage aligns with all applicable laws and standards.
Ultimately, AI-augmented financial analysis isn’t just about incremental improvements or cost-cutting. It represents a fundamental shift, offering transformative potential to enhance the quality of insight, uncover hidden opportunities, and guard more effectively against emerging risks. My perspective, forged through years of observing system deployments, is that the most effective path involves combining a solid, adaptable architecture with a relentless focus on practical application. This ensures AI delivers tangible benefits and builds crucial organizational capacity for the future.
For professional connections and further discussion, you can find me on LinkedIn.