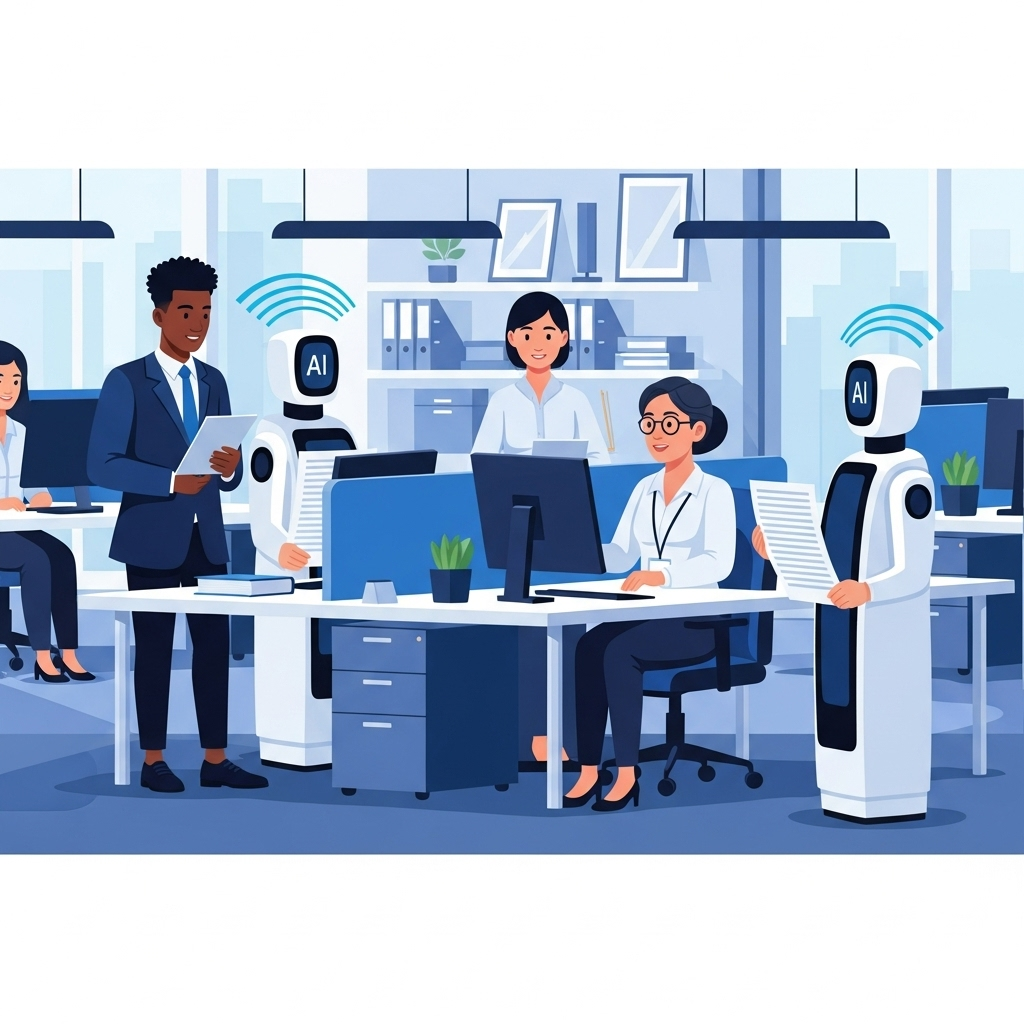
Table of Contents
Artificial intelligence is fundamentally transforming accounting and finance functions, moving beyond simple automation toward true cognitive assistance. While robotic process automation (RPA) has long addressed structured tasks, emerging AI agents now handle increasingly complex accounting processes that demand judgment and contextual understanding. This shift dramatically reshapes the accounting profession, calling for new skills and organizational models. Don’t you think that’s a profound change?
Beyond RPA: The Cognitive Automation Leap
Traditional automation in accounting focused primarily on rule-based processes with structured inputs and clear decision trees. AI agents represent a significant evolution by incorporating multiple capabilities:
Multi-system Integration: Unlike single-purpose tools, modern AI agents interact across enterprise systems, extracting and combining information from ERP platforms, banking systems, supporting documentation, and communication channels. (It’s a bit like a digital octopus, reaching into various data pools.)
Context-aware Processing: Advanced agents understand transaction context beyond simple classification rules. They can interpret email exchanges, recognize unusual patterns, and connect related transactions across different systems.
Judgment Simulation: While not replacing human judgment, AI agents increasingly simulate basic professional judgment through large language models grounded in accounting knowledge bases and organizational context.
Continuous Learning: Unlike static automation, AI agents evolve through feedback loops, improving accuracy over time by incorporating how finance professionals handle exceptions.
These capabilities enable automation of previously untouchable accounting processes, ones that require professional interpretation and judgment.
Transforming Core Accounting Processes
Several accounting domains demonstrate particularly dramatic transformation:
Month-end Close: Traditional close processes involve numerous reconciliations, accruals, and reviews. AI agents now automate many of these activities by learning pattern recognition for account fluctuations, preparing standard journal entries with appropriate supporting documentation, and flagging only genuine anomalies for human review. (Imagine the hours saved!)
Audit Support: AI agents facilitate both internal and external audits by retrieving requested samples, assembling supporting documentation, explaining transaction rationales, and providing explanations for fluctuations in preprogrammed formats.
Accounts Payable: Beyond basic matching, advanced agents now handle exception management, communicating directly with vendors to resolve discrepancies, routing approval requests with appropriate context, and learning organization-specific processing rules.
Financial Analysis: Agents increasingly conduct first-level variance analysis, generating narrative explanations for budget deviations and recommending potential corrective actions based on historical patterns.
In each case, AI doesn’t simply execute predefined steps but adapts to variations and exercises limited professional judgment within defined parameters. This is a key differentiator from older tech.
The Evolving Accounting Role
This transformation fundamentally changes what finance professionals do. Rather than disappearing, accounting roles evolve toward higher-value activities:
From Transaction Processing to Exception Handling: Accountants shift from routine processing to managing complex exceptions that require genuine professional judgment that AI cannot yet replicate.
From Data Compilation to Insight Generation: With reports and analyses auto-generated, finance professionals focus on deriving strategic insights and recommendations from financial results.
From Rule Execution to Rule Design: Accounting teams increasingly design the parameters and frameworks within which AI agents operate, rather than executing those rules themselves.
From System Users to System Supervisors: Finance professionals transition from using systems directly to supervising AI agents, reviewing outputs, and providing feedback to improve performance.
This evolution demands significant skill development across technical, analytical, and interpersonal dimensions. Finance professionals need greater understanding of AI capabilities and limitations, stronger analytical skills to interpret AI-generated insights, and enhanced communication abilities to translate financial information for non-financial stakeholders. It’s a whole new ballgame.
Implementation Models and Considerations
Organizations implement accounting AI through several models, each with distinct implications:
Centralized AI Center of Excellence: Some organizations establish dedicated teams that develop, deploy, and maintain finance AI agents. This approach ensures consistent implementation and specialized expertise but may create bottlenecks.
Embedded Finance Technology Teams: Other organizations embed AI specialists directly within finance functions, creating closer alignment with accounting processes but potentially fragmenting technical expertise.
Vendor-Driven Solutions: Many organizations leverage pre-built AI agents from financial software vendors, reducing implementation complexity but potentially limiting customization.
Hybrid Approaches: Leading organizations often combine these models, using vendor solutions for common processes while building custom agents for organization-specific needs. What’s the best fit for any given company? That often depends on their existing structure and strategic priorities.
Implementation success depends less on the specific model than on several critical factors:
Leadership Alignment: Finance leadership must actively champion AI adoption while establishing clear boundaries for appropriate automation.
Process Standardization: AI agents perform better against standardized processes. Organizations often need to streamline processes before effective agent deployment.
Data Quality Governance: AI agents depend heavily on data quality. Successful implementations establish robust data governance processes to ensure reliable inputs.
Change Management: Perhaps most critically, organizations must help accounting professionals navigate the transition from process execution to agent supervision.
Ethical and Control Considerations
The introduction of AI agents in accounting raises important ethical and control considerations:
Accountability Frameworks: Organizations must define clear responsibility boundaries between agents and humans, particularly regarding financial statement preparation and certification.
Control Environment: Internal control frameworks require adaptation to address risks specific to AI agents, including potential biases, systematic errors, and security vulnerabilities.
Transparency Requirements: Finance leaders should establish explainability standards for AI agent decisions, particularly for those affecting financial reporting or compliance matters.
Professional Standards: Accounting standard-setters and professional bodies continue developing guidance on appropriate use of AI in financial reporting and audit processes.
These considerations require thoughtful governance models that balance innovation with appropriate risk management.
Looking Forward
The trajectory is clear: AI agents will continue expanding their role in accounting and finance functions. Organizations that proactively manage this transition position their finance teams as strategic business partners rather than transaction processors.
For finance professionals, this evolution represents both challenge and opportunity. Those who develop AI supervision skills, enhance their analytical capabilities, and strengthen their strategic perspective will thrive. Those who remain focused solely on transaction processing face diminishing opportunities.
The most successful organizations view this transition not simply as a cost-reduction exercise but as a fundamental transformation of the finance function’s value proposition. My research certainly points in this direction.