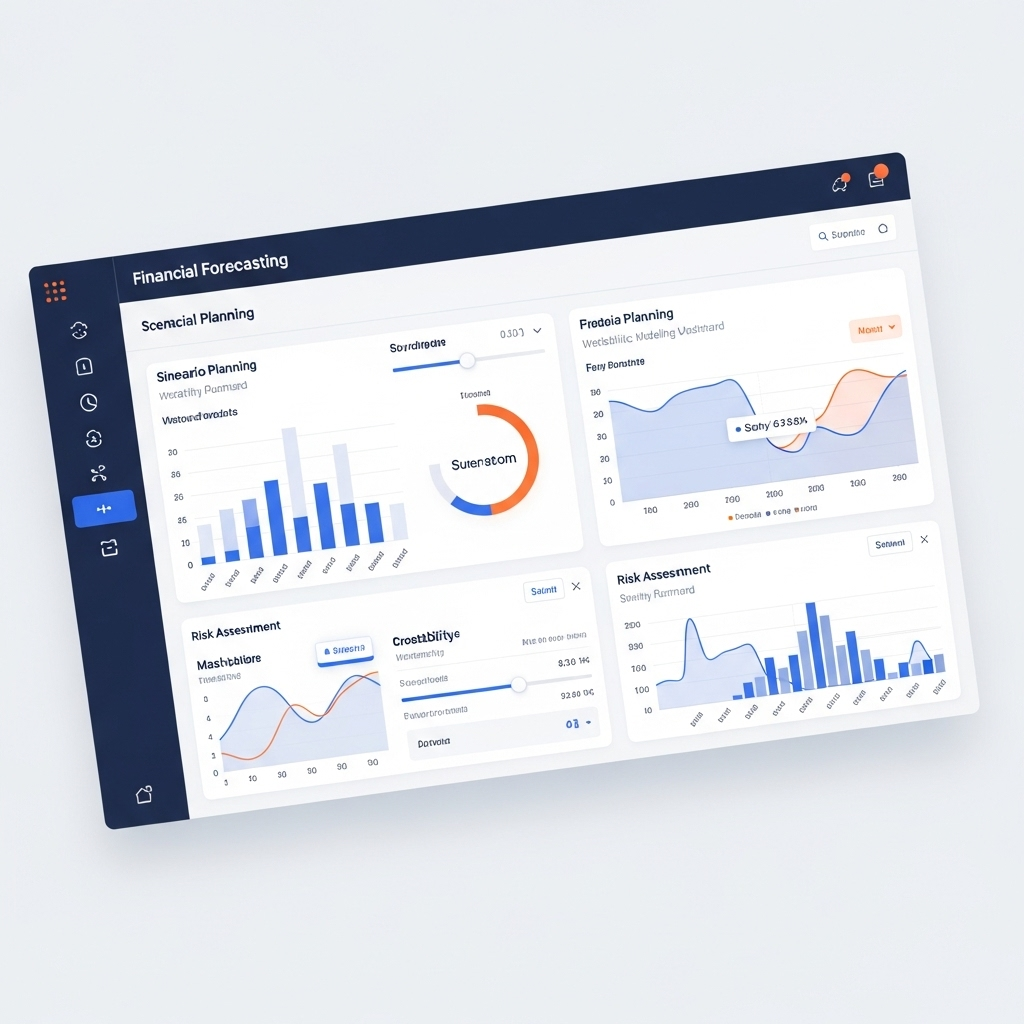
Table of Contents
Traditional financial forecasting methods often produce point estimates that create an illusion of certainty. It’s a common trap, isn’t it? Industry analysis repeatedly shows how this approach breaks down, especially in volatile economic environments. Modern forecasting techniques, thankfully, explicitly acknowledge uncertainty, paving the way for more robust decision-making when conditions are ambiguous.
The Problem with Point Estimates
Standard financial forecasts typically give you single-value projections (revenue will be $X, costs will be $Y, resulting in a profit of $Z. This approach fundamentally misrepresents the probabilistic nature of business outcomes. Research across multiple industries indicates that even sophisticated forecasting models rarely achieve better than 70-80% accuracy for key financial metrics. That’s a pretty wide margin for error.
This gap between projection and reality creates significant operational challenges. Organizations end up making resource allocation decisions based on false precision, which often leads to inefficient capital deployment and frequent, disruptive mid-period adjustments.
Embracing Uncertainty through Range Forecasting
Leading financial planning practices now build explicit uncertainty into their forecasting methodologies. Range forecasting is the simplest way to do this. Instead of projecting single values, analysts define reasonable boundaries for each metric. What does an effective range forecast usually include? You’re looking at a base case (the most likely outcome), along with upper and lower boundaries representing reasonable extremes. It’s also vital to identify key inflection points (where strategy might need to change) and be crystal clear about the critical assumptions) those variables with the highest impact on outcomes. This approach acknowledges inherent uncertainty while still providing actionable guidance.
Scenario-Based Planning
More sophisticated organizations take it a step further by implementing full scenario modeling within their financial planning processes. This methodology explores multiple potential futures rather than trying to predict a single one. So, how does it work?
Effective scenario planning involves several key steps:
- Identifying key uncertainties that could materially impact financial outcomes.
- Creating distinct, plausible narratives around how these uncertainties might unfold.
- Modeling the financial impacts across each of these unique scenarios.
- Developing contingency plans and clear decision triggers for when to activate them.
Unlike simple sensitivity analysis, scenario planning explores how multiple variables might change together in coherent patterns. This is crucial because it reveals systemic relationships that single-variable analysis often misses.
Probabilistic Forecasting Methods
Organizations with the most advanced financial planning capabilities often employ probabilistic forecasting methods. These techniques don’t just give point estimates or simple ranges; they express outcomes as probability distributions. Monte Carlo simulation is the most widely adopted probabilistic approach. This method involves defining probability distributions for key inputs, then running thousands of simulations with randomly selected values from those distributions. The output? Probability distributions for outcomes, which help identify the likelihood of achieving various targets. The beauty of this is that decision-makers get a much more nuanced understanding of potential outcomes, including those pesky tail risks that traditional methods frequently overlook.
Implementation Challenges
Transitioning to uncertainty-focused forecasting isn’t always a walk in the park. There are technical hurdles, like data requirements, modeling complexity, and integration with existing systems. However, it’s often organizational resistance that presents a more significant barrier. (People love certainty, even if it’s false!) Executive teams accustomed to deterministic forecasts frequently struggle with probabilistic thinking. Successfully implementing these methods requires a concerted effort. This includes educational initiatives on probability concepts and using visualization techniques that communicate uncertainty effectively. Decision processes also need to be adapted for probabilistic inputs, and critically, performance evaluation metrics should reward forecast quality and insight, rather than just pinpoint accuracy.
Organizations that manage to overcome these challenges often develop a significant competitive advantage. Why? Better capital allocation decisions and more agile responses to changing conditions.
Technological Enablers
Modern forecasting platforms are increasingly incorporating native support for uncertainty modeling. Instead of requiring specialized statistical knowledge, these tools provide accessible interfaces for scenario planning and probability-based projections. Advanced systems leverage features like automated scenario generation, AI-assisted assumption setting, and real-time data integration for continuous reforecasting. They also offer visual interfaces for exploring probability distributions and collaborative tools for cross-functional input. These capabilities are democratizing access to sophisticated forecasting methods, extending their benefits beyond specialized financial analysis teams.
The Future of Financial Forecasting
The trajectory of financial forecasting clearly points toward increasingly adaptive, probability-based approaches. Organizations clinging to traditional point-forecast methodologies will likely find themselves at a competitive disadvantage as business environments become more volatile and interconnected. What are forward-thinking finance teams doing? They’ve begun implementing continuous forecasting processes that constantly update projections based on new information. This approach abandons artificial calendar-based cycles in favor of event-triggered revisions, further improving forecast relevance.
Embracing uncertainty doesn’t mean abandoning the pursuit of accuracy. Rather, it acknowledges the inherent limitations of prediction while providing far more comprehensive information for decision-makers navigating today’s complex business environments.